脏数据
- 网络Dirty data;Out-of-date data
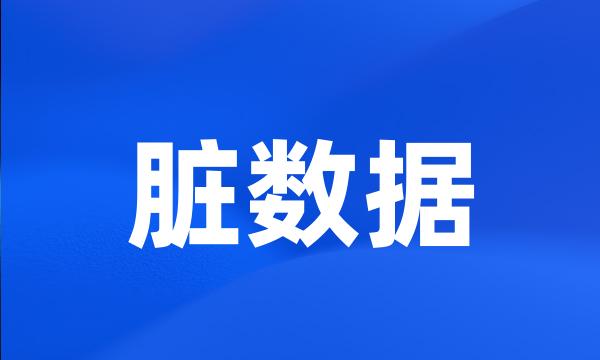
-
文中提出了基于改进ART2网络的脏数据辨识与调整模型。
A model for dirty data identification and justification is put forward based on the modified ART2 network .
-
它并不等于fsync()函数&也不是请求同步脏数据。
It is not equivalent to fsync () & it 's not a request to sync dirty data .
-
DB2页面清理器实际上要负责将脏数据或不需要的数据从缓冲池转移到磁盘。
DB2 page cleaners essentially have the responsibility of removing dirty or unneeded data from the bufferpools to disk .
-
数据库读写器(DBWr)&异步地将缓冲区的脏数据写到物理数据文件中。
Database Writer ( DBWr ) & Writes to physical data files the dirty bits of buffer asynchronously .
-
一种基于交替投影的脏数据处理方法
A new dirty data processing method based on alternative projection
-
基于数据挖掘的电力负荷脏数据动态智能清洗
Dynamic Intelligent Cleaning for Dirty Electric Load Data Based on Data Mining
-
如果有丢失的或脏数据,您将如何进行呢?
If there is missing or dirty data , how will you proceed ?
-
基于日志的脏数据检测与恢复
Log Based Data Corruption Detection and Recovery
-
除了上述问题之外,与“脏数据”相关的问题也总是存在。
Beyond the issues above are the ever present problems associated with " dirty data " .
-
这就是与干净数据相比,“脏数据”的开销更小的原因所在。
This is why there is less overhead for dirty data as compared to clean data .
-
结果表明,该清洗规则对错误、丢失、冗余等“脏数据”的识别率均在90%以上。
Results show that the recognition rates of " dirty data " is up to90 % .
-
特别对于日志的预处理中的问题,提出了日志的脏数据和噪声数据两个概念,并且对这两个概念进行了详细的阐述并做了比较。
We bring forward two definitions including dirty data and noise data , in the pretreatment process .
-
首先,对原始的日志文件进行了必要的预处理,清除脏数据。
First , preprocessing to the primitive log files were carried on to clear the dirty data .
-
“脏数据”的生成方法与干净数据的生成方法类似。
It was generated using an approach similar to the approach used to generate the clean data .
-
脏数据处理的过程就是对于含有脏数据的负荷曲线模式的辨识过程。
The process of dirty data processing is the recognition process of load curve pattern containing dirty data .
-
而各种数据,包括脏数据,清洗后的数据,验证后的结果,清洗过程中要用到的数据字典等等都数据信息范围。
Moreover , dirty data , cleaned data , result of validation and data dictionary used by cleaning procedure are all information .
-
构建了清洗规则定制模块,解决了单记录型脏数据的清洗问题;研究了缺损数据和相似重复记录两类常见多记录型脏数据的清洗策略;
As to multi-record one , the thesis studies the cleaning strategy of two common kinds : incomplete data and duplicate records .
-
最终说明了所有过滤条件都是有效而且高效的,检测系统检测的结果也和预先引入的脏数据一致。
Eventually note that the filters are effective and efficient , the result of the detection system are identical to the pre-test results .
-
合并意味着如果相同的记录被更新,或者在缓冲区内被多次标记为脏数据,则只保证最后一次更新。
Conflation means if the same record is updated or dirtied multiple times within the buffering period then it only keeps the last update .
-
详细而准确的元数据对于数据仓库的创建、数据加载、运行维护、清理脏数据等工作都必不可少。
Detailed and exact metadata is absolutely necessarily for the creation , the data loading , the data cleaning and regular maintenance of a data warehouse .
-
如果无法在客户的数据源中纠正丢失的数据或脏数据,什么业务规则将用于纠正数据呢?
If the missing data or dirty data cannot be corrected in the customer ? s data sources , what business rules will be used to correct the data ?
-
将脏数据按照清洗方式的差异划分为单记录型和多记录型脏数据两类,并提出了解决两类脏数据的清洗策略。
Categorized dirty data into single-record one and multi-record one according to the difference in cleaning measures , and then solved the cleaning of single-record dirty data by utilizing cleaning rules .
-
由于性能和其他要求,存在一类特殊的应用,它们要求大批量数据常驻内存,并直接在内存中对数据进行存取访问,增加内存中产生脏数据的可能性。
For better performance and other purposes , some special applications have direct access to data which resides in the main memory , as a result of which , the risk of data being corrupted due to application default is increased .
-
针对工作流的长时间作业的特点,论文提出了一个基于向后恢复的工作流事务模型,可以避免读脏数据以及简化事务冲突分析,实现高效率的细粒度的工作流事务管理。
Aiming at the characteristics of long-term task in workflow , a workflow transaction model based on backward recovery was surveyed , which could avoid the situation of reading dirty data , simplify confliction analysis , and provide efficient workflow transaction management .
-
论文研究数据集成过程中脏数据和数据源异构问题的解决方法,重点研究了数据清洗策略及其相关算法,为消除脏数据、保证集成数据的质量提供了一套通用的解决方案。
The thesis studies the problems to be solved in the process of heterogeneous data source integration , focusing on the data cleaning strategy and the algorithm involved . It provides a universal solution to clean dirty data for guaranteeing the quality of integrated data .
-
如果我们两个都有,当对所有行都要操作时,我们可以在只读窗口中运行UR,而在需要进行维护并且避免受到“脏”数据影响时,运行CS。
If we have both , we could run with UR during read-only windows when all of the rows are committed and run with CS when maintenance is being performed and we need to be protected from " dirty " data .
-
Fowler和Christakis参考伯明翰心脏研究中心的一项心血脏研究数据绘出了4739个个体的社会关系网图,这项研究的参与者罗列出了他们最亲近的朋友,家庭成员和邻居的相关信息,并组合成了超过50000条的社会链。
Fowler and Christakis were able to map the social networks of 4739 individuals with data from the Framingham Heart Study , an ongoing cardiovascular study . Participants in that study listed contact information for their closest friends , family members and neighbors , connecting the pair of researchers to more than 50000 social ties .
-
如果有丢失数据或脏(dirty)数据,您的客户是否可以在数据源中进行纠正呢?
If there is any missing data or dirty data , can your customer correct this in the data sources ?
-
换句话说,将把数据缓冲区的所有脏位刷新到数据文件。
In other words , all dirty bits of the data buffer will be flushed to the data files .