贝叶斯概率
- 网络Bayesian probability
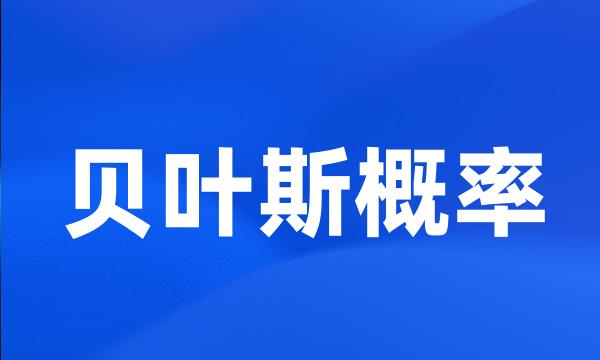
-
在融合过程中以贝叶斯概率模型修正先验概率。
The prior probability is amended by Bayesian probability model in the fusion process .
-
仿真结果证明,这种采用改进的贝叶斯概率加权多模型预测控制方法,具有很好的控制品质。
The simulation results proved that the Bayesian probability weighted multi-model predictive control method have good quality control .
-
基于BP神经网络的贝叶斯概率水文预报模型
Bayesian probabilistic forecasting model based on BP ANN
-
应用机器学习技术,如K-邻近法、贝叶斯概率模型等已经实现了Web文档半自动和自动分类。
The application of well-known machine learning technology , including K-Nearest Neighbor , Naive Bayes and etc. , have brought out the automatic and semi-automatic Web classification .
-
最后,本文通过实际的案例&电脑拆卸来证实将Petri网和贝叶斯概率推理应用到动态业务流程优化中的可行性。
Finally , the paper confirms the feasibility of applying Petri net and Bayesian inference to the dynamic optimization of business processes through actual case-PC Demolition .
-
在控制系统中,将贝叶斯概率引入到模糊RBF神经网络中,增强了系统的推理能力,提高了飞机各个航道位置的模拟伺服精度。
In the control system , Bayes probability is introduced in the fuzzy RBF neural network and it intensity the inference ability and increase the servo precision .
-
贝叶斯概率与元胞自动机的非线性转换规则
Nonlinear Transition Rules of Urban Cellular Automata Based on a Bayesian Method
-
基于贝叶斯概率模型的邮件过滤算法探讨
Research on mail filter algorithm based on bayes probability model
-
贝叶斯概率水文预报系统在中长期径流预报中的应用
Application of the Bayesian statistic hydrological forecast system to middle-and long-term runoff forecast
-
贝叶斯概率洪水预报系统
Probabilistic Flood Forecasting System Based on Bayesian Method
-
贝叶斯概率图像自动分割研究
A Study on Bayesian Probabilistic Image Automatic Segmentation
-
以贝叶斯概率和贝叶斯网络基本理论为基础,主要研究了贝叶斯网络的结构学习和使用贝叶斯网络进行知识发现和决策支持的方法。
The paper mainly studies structure learning of Bayesian networks for knowledge discovery and decision supporting system .
-
另外,提出了分两阶段由粗到精的跟踪方法和基于贝叶斯概率理论的多个目标对象发生遮挡时的处理方法。
Otherwise , we propose two phases tracking strategy , which means coarse-to-fine idea and the solution of multi-objects occlusion based on Bayesian framework .
-
作者通过分析研究垃圾邮件关键词的统计概率分布,提出了基于贝叶斯概率模型的邮件过滤算法,并对该算法的合理性和复杂度进行了分析。
In this model , the author puts forward the filter algorithm based on Bayes distributing by researching the statistical distributing of the spam keys .
-
因此,本文采用改进的贝叶斯概率加权多模型预测控制策略控制整个拉晶过程的热场温度。
So this paper proposed using improved Bayesian probability weighted multi-model predictive control algorithm to control the thermal field temperature of all crystal pulling process .
-
基于已有的道路交通事故统计资料,应用动态聚类分析方法和贝叶斯概率模型,对事故多发点的鉴定方法进行了探讨。
On the basis of road accident records , adopting stepwise cluster analysis and Bayesian probabilistic model , this paper investigates the method of identification of road accident blackspots .
-
文中将决策树方法与贝叶斯概率分析相结合,结合实例提出了贝叶斯方法在房地产投资风险决策的应用方法和相关决策规则的选择思路。
Using the methods of BAYES and decision tree , this paper introduced the application method and relational decision rules of BAYES in real investment risk decision-making by a case .
-
本文采用统计分析的方法建立了理想状态下和贝叶斯概率模型有一定偏差的贝叶斯优化算法的数学模型;并在此基础上用统计分析的方法证明了贝叶斯优化算法的收敛性。
The ideal mathematic model of Bayesian optimization algorithm is set up based on probability analysis . Then it is found that the algorithm will converge to the global optimum .
-
贝叶斯概率理论是数学概率论的一个分支,它通过将先验知识和观测事件结合起来而允许我们对于现实世界中的不确定性建模并进行感兴趣的推理。
Bayesian probability theory is a branch of mathematical probability theory that allows one to model uncertainty about the world and outcomes of interest by combining common-sense knowledge and observational evidence .
-
多线索融合的方法可以显著提高目标跟踪的性能,本文提出了一种贝叶斯概率框架下多线索融合的判别式视频目标轮廓跟踪算法。
It is well known that combining multiple cues can significantly improve the performance of a visual object tracker . A discriminative video object contour tracking algorithm using multi-cue under Bayesian inference is proposed .
-
其次,应用贝叶斯概率理论对基于信号强度经验模型的定位问题进行建模,通过实验研究了信号强度的分布特征,证明了当传输距离固定时节点接收到的信号强度值基本服从正态分布。
Secondly , we modeled the localization problem with Bayesian Probability Theory , studied the distribution of signal strength through experiments , and then proved that the RSSI with fixed distance is normally distributed .
-
贝叶斯概率框架是一种将先验概率转化为后验概率的理论框架,通过形式化的图像分类概率框架可以将低级图像特征映射到已有的高层语义。
Bayesian probability framework is a theory to transfer a priori probability to posterior probability . This thesis provides a formal Bayesian framework for image classification problems , which maps the low-level image features to the intrinsic high-level semantics .
-
证据理论作为一种不精确推理理论,不仅有处理未知信息的特性,而且其主要特点满足比贝叶斯概率论更弱的条件,具有直接表达不确定和不知道的能力。
D-S theory ( Dempster-Shafer theory ), as a kind of inexact reasoning theory , the main characteristics of it satisfy the weaker condition than Bayesian probability theory.D-S theory has the ability to deal with unknown information and express the uncertain or unknown information .
-
贝叶斯最小概率模式识别在水下V形焊缝识别中光斑去除的应用
Application of mode detection based on the minimum probability of Bayes in faculae removal in underwater V-groove weld
-
主体关联性对贝叶斯推理概率估计的影响
A Study on the Influence of Subject Relevancy on Bayesian Reasoning
-
采用人工智能中的贝叶斯网络概率传播算法对串行译码方法的合理性给出了证明。
We also use Pearl 's probability propagation algorithm to show the appropriateness of the serial decoding method .
-
在以声学模型为中心的邻接空间中计算贝叶斯预测概率密度值,作为观察概率输出分值进行识别。
This approach uses neighborhood space of each underlying model to produce Bayesian predictive density as observation probability density .
-
最近邻准则是一种次最优准则,当样本数目很大时,最近邻准则的错误率不会超过贝叶斯错误概率的2倍。
The misclassification probability of the nearest neighbor decision rule won 't exceed 2 times of that of Bayes decision rule when the sample number is very large .
-
本文主要涉及的不确定推理模型包括主观贝叶斯的概率推理模型,可信度理论推理模型,证据理论及其改进推理模型以及神经网络推理模型。
In the paper , the models of uncertain reasoning are focused , such as the reasoning model of Bayes probability , Reliability theory , D-S evidence theory and Neural Network .
-
介绍贝叶斯网络概率推理方法,包括贝叶斯网络基本概念和性质,贝叶斯网络建模及目前常用贝叶斯精确概率推理方法。第三,对软件项目风险控制与决策方法进行研究。
Then the method of Bayesian Networks probability inference was introduced , including the conception , features , modeling and the precise probability reasoning method . Thirdly , this paper also involved the software project risk control .